github链接:https://github.com/ZHKKKe/MODNet
一、安装运行环境
conda create -n modent python=3.8
conda activate modent
pip install torch-1.10.0+cu113-cp38-cp38-win_amd64.whl
pip install torchvision-0.11.0+cu113-cp38-cp38-win_amd64.whl
pip install opencv-python -i https://mirror.baidu.com/pypi/simple
pip install onnx==1.8.1 -i https://mirror.baidu.com/pypi/simple
pip install onnxruntime==1.6.0 -i https://mirror.baidu.com/pypi/simple
pip install tqdm -i https://mirror.baidu.com/pypi/simple
二、运行
1、第一种抠图
创建inference2.py
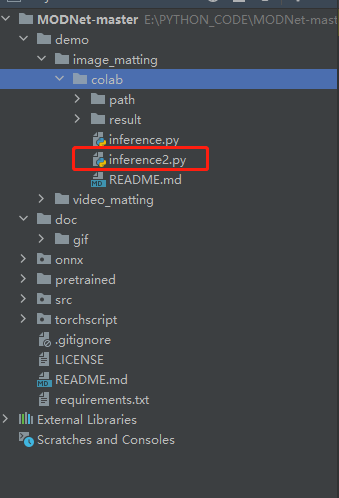
代码如下:
import os
import sys
import argparse
import numpy as np
from PIL import Image
import torch
import torch.nn as nn
import torch.nn.functional as F
import torchvision.transforms as transforms
from src.models.modnet import MODNet
if __name__ == '__main__':
# define cmd arguments
parser = argparse.ArgumentParser()
parser.add_argument('--input_path', default='E:/PYTHON_CODE/MODNet-master/demo/image_matting/colab/path/', type=str, help='path of the input image (a file)')
parser.add_argument('--output_path', default='./result', type=str,
help='paht for saving the predicted alpha matte (a file)')
parser.add_argument('--ckpt_path', default='E:/PYTHON_CODE/MODNet-master/pretrained/modnet_photographic_portrait_matting.ckpt', type=str, help='path of the ONNX model')
args = parser.parse_args()
# check input arguments
if not os.path.exists(args.input_path):
print('Cannot find input path: {0}'.format(args.input_path))
exit()
if not os.path.exists(args.output_path):
print('Cannot find output path: {0}'.format(args.output_path))
exit()
if not os.path.exists(args.ckpt_path):
print('Cannot find ckpt path: {0}'.format(args.ckpt_path))
exit()
# define hyper-parameters
ref_size = 512
# define image to tensor transform
im_transform = transforms.Compose(
[
transforms.ToTensor(),
transforms.Normalize((0.5, 0.5, 0.5), (0.5, 0.5, 0.5))
]
)
# create MODNet and load the pre-trained ckpt
modnet = MODNet(backbone_pretrained=False)
modnet = nn.DataParallel(modnet).cuda()
if torch.cuda.is_available():
modnet = modnet.cuda()
weights = torch.load(args.ckpt_path)
else:
weights = torch.load(args.ckpt_path, map_location=torch.device('cpu'))
modnet.load_state_dict(weights)
modnet.eval()
# inference images
im_names = os.listdir(args.input_path)
for im_name in im_names:
print('Process image: {0}'.format(im_name))
# read image
im = Image.open(os.path.join(args.input_path, im_name))
# unify image channels to 3
im = np.asarray(im)
if len(im.shape) == 2:
im = im[:, :, None]
if im.shape[2] == 1:
im = np.repeat(im, 3, axis=2)
elif im.shape[2] == 4:
im = im[:, :, 0:3]
im_org = im # 保存numpy原始数组 (1080,1440,3)
# convert image to PyTorch tensor
im = Image.fromarray(im)
im = im_transform(im)
# add mini-batch dim
im = im[None, :, :, :]
# resize image for input
im_b, im_c, im_h, im_w = im.shape
if max(im_h, im_w) < ref_size or min(im_h, im_w) > ref_size:
if im_w >= im_h:
im_rh = ref_size
im_rw = int(im_w / im_h * ref_size)
elif im_w < im_h:
im_rw = ref_size
im_rh = int(im_h / im_w * ref_size)
else:
im_rh = im_h
im_rw = im_w
im_rw = im_rw - im_rw % 32
im_rh = im_rh - im_rh % 32
im = F.interpolate(im, size=(im_rh, im_rw), mode='area')
# inference
_, _, matte = modnet(im.cuda() if torch.cuda.is_available() else im, True)
# resize and save matte
matte = F.interpolate(matte, size=(im_h, im_w), mode='area')
matte = matte[0][0].data.cpu().numpy()
matte_name = im_name.split('.')[0] + '.png'
Image.fromarray(((matte * 255).astype('uint8')), mode='L').save(os.path.join(args.output_path, matte_name))
matte_org = np.repeat(np.asarray(matte)[:, :, None], 3, axis=2) # 扩展到 (1080, 1440, 3) 以便和im_org计算
foreground = im_org * matte_org + np.full(im_org.shape, 255) * (1 - matte_org) # 计算前景,获得抠像
fg_name = im_name.split('.')[0] + '_fg.png'
Image.fromarray(((foreground).astype('uint8')), mode='RGB').save(os.path.join(args.output_path, fg_name))
2、第二种实时抠视频
指定模型的绝对路径
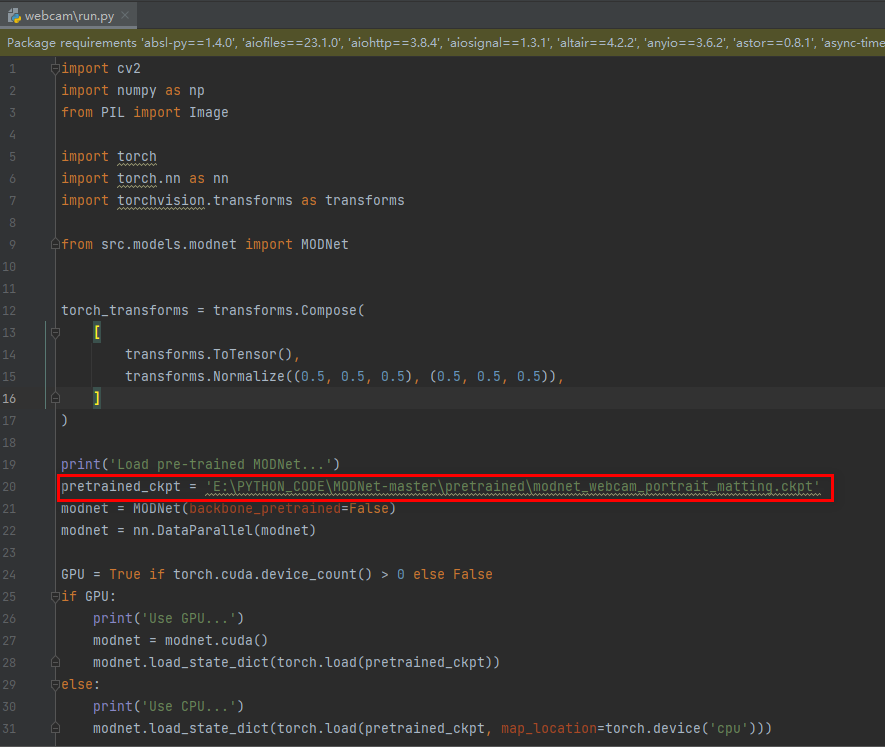
模型位置如下:
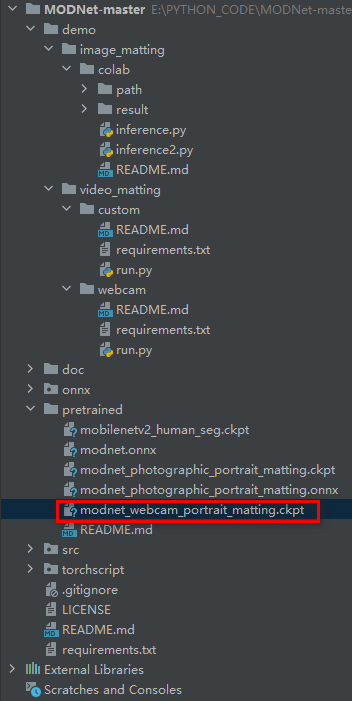
运行 run.py文件
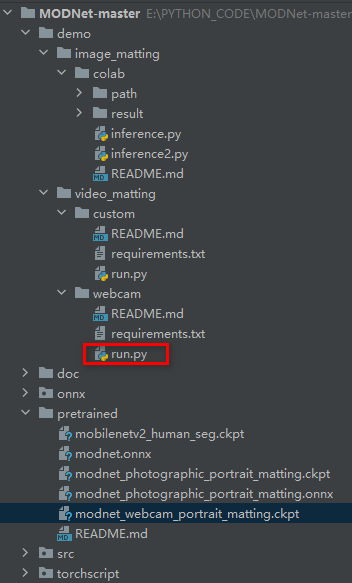